The main focus of relational learning for knowledge graph completion (KGC) lies in exploiting rich contextual information for facts. Many state-of-the-art models incorporate fact sequences, entity types, and even textual information. Unfortunately, most of them do not fully take advantage of rich structural information in a KG, i.e., connectivity patterns around each entity. In this paper, we propose a context-aware convolutional learning (CACL) model which jointly learns from entities and their multi-hop neighborhoods. Since we directly utilize the connectivity patterns contained in each multi-hop neighborhood, the structural role similarity among entities can be better captured, resulting in more informative entity and relation embeddings. Specifically, CACL collects entities and relations from the multi-hop neighborhood as contextual information according to their relative importance and uniquely maps them to a linear vector space. Our convolutional architecture leverages a deep learning technique to represent each entity along with its linearly mapped contextual information. Thus, we can elaborately extract the features of key connectivity patterns from the context and incorporate them into a score function which evaluates the validity of facts. Experimental results on the newest datasets show that CACL outperforms existing approaches by successfully enriching embeddings with neighborhood information.
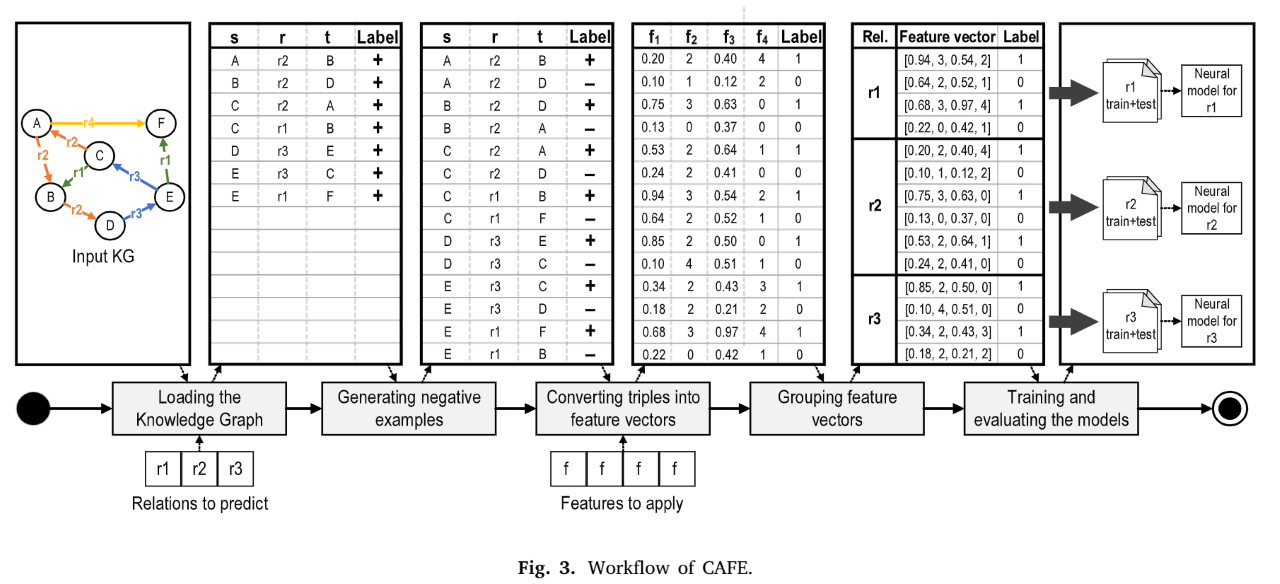
Related Articles
2021
CAFE: Knowledge graph completion using neighborhood-aware features Journal Article
In: Engineering Applications of Artificial Intelligence, vol. 103, pp. 104302, 2021, ISSN: 0952-1976.
How to use CAFE
You can find CAFE’s full documentation on its Github link above.